

With the new vacancy, we added another beginner-friendly tome with Elementary Statistics. We also removed Statistics for Management and Economics, which is still a solid text, however, isn't as compelling as Statistics for Business & Economics, which covers many of the same bases. It's a good supplement to get you thinking outside the box for class and is ideal for anyone toying with entering this field. It's also meant to empower the everyday person to know when they are being confronted with biased data that lacks context, a skill anyone in the information age should welcome. Written with enthusiasm by an expert, this volume takes extremely relatable and interesting real-life scenarios and shows readers how statistics affect them. We still wanted to have something on deck to cater to laypeople, though, and so brought on The Art of Statistics. It's supposed to track with most first-semester intro courses, however, many students find they still need another textbook. We said goodbye to Statistics For Dummies, which can sometimes manage to be confusing while simultaneously not going deep enough into the concepts. We also updated Statistics for People Who (Think They) Hate Statistics to its newest seventh edition. (h) In this data set, how many of the suburbs average more than seven rooms per dwelling? More than eight rooms per dwelling? Comment on the suburbs that average more than eight rooms per dwelling.Not too much has changed in this category since our last update, but we decided to prune our list a bit in order to bring on a few new relevant selections. (g) Which suburb of Boston has lowest median value of owneroccupied homes? What are the values of the other predictors for that suburb, and how do those values compare to the overall ranges for those predictors? Comment on your findings. (f) What is the median pupil-teacher ratio among the towns in this data set? (e) How many of the suburbs in this data set bound the Charles river?
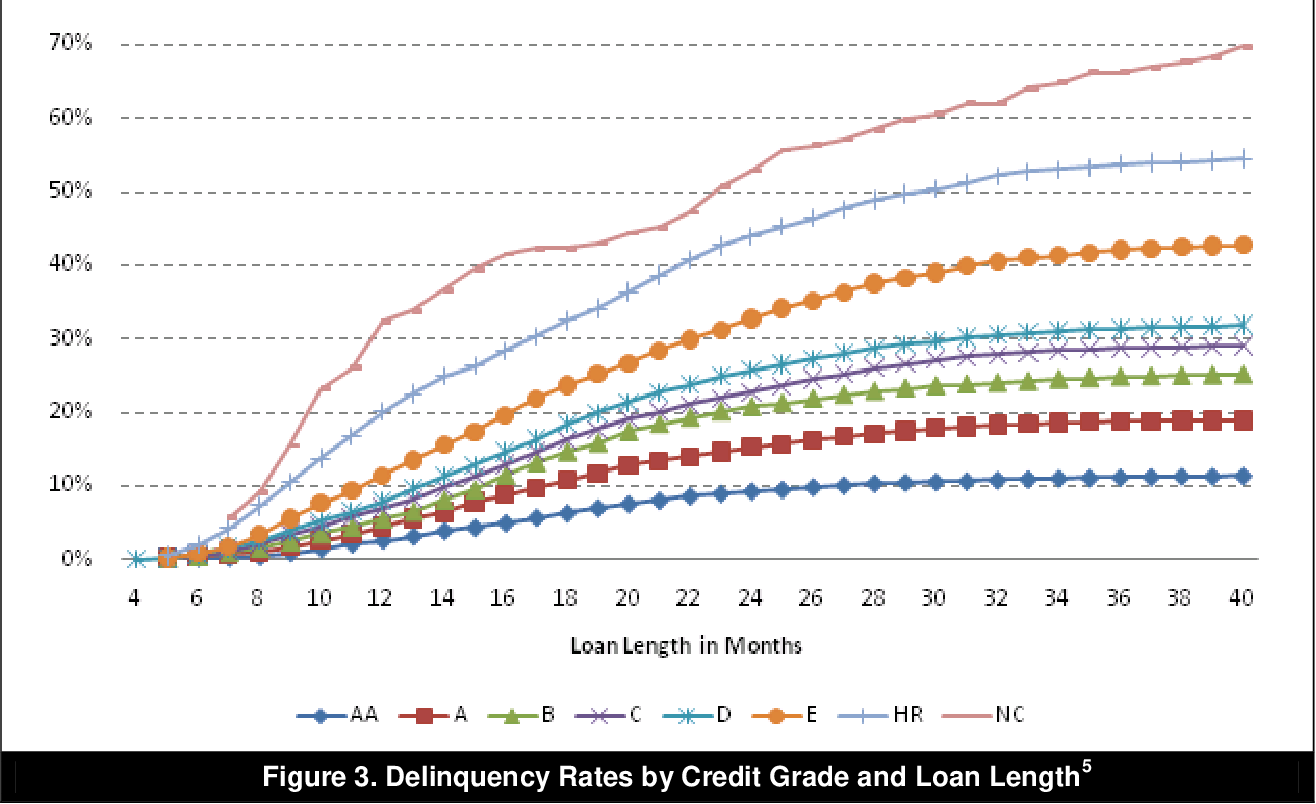
(d) Do any of the suburbs of Boston appear to have particularly high crime rates? Tax rates? Pupil-teacher ratios? Comment on the range of each predictor. (c) Are any of the predictors associated with per capita crime rate? If so, explain the relationship. (b) Make some pairwise scatterplots of the predictors (columns) in this data set. How many rows are in this data set? How many columns? What do the rows and columns represent? Now the data set is contained in the object Boston.
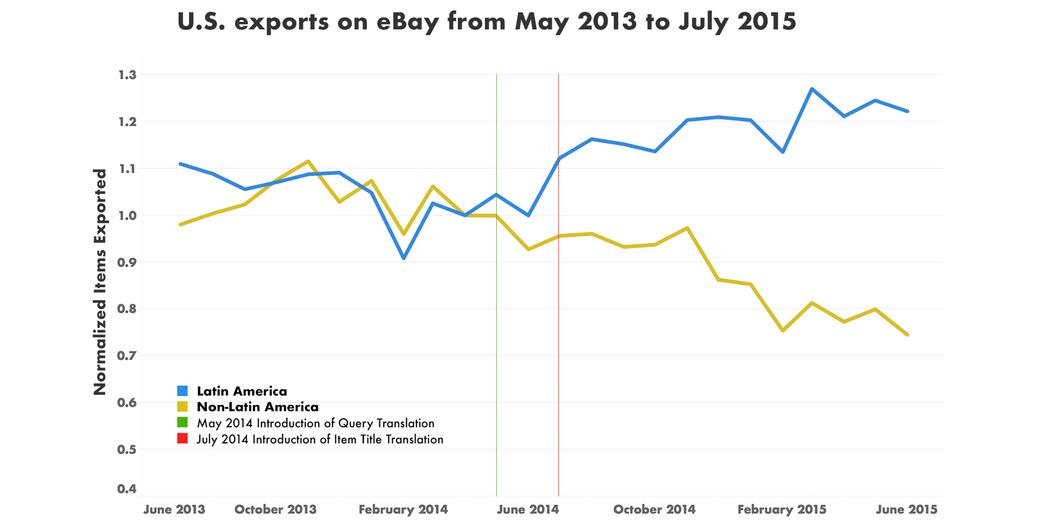
You should see that there is now a row.names column with the name of each university recorded. However, it may be handy to have these names for later. We don’t really want R to treat this as data. You should notice that the first column is just the name of each university. (b) Look at the data using the fix() function. Make sure that you have the directory set to the correct location for the data. (a) Use the read.csv() function to read the data into R.
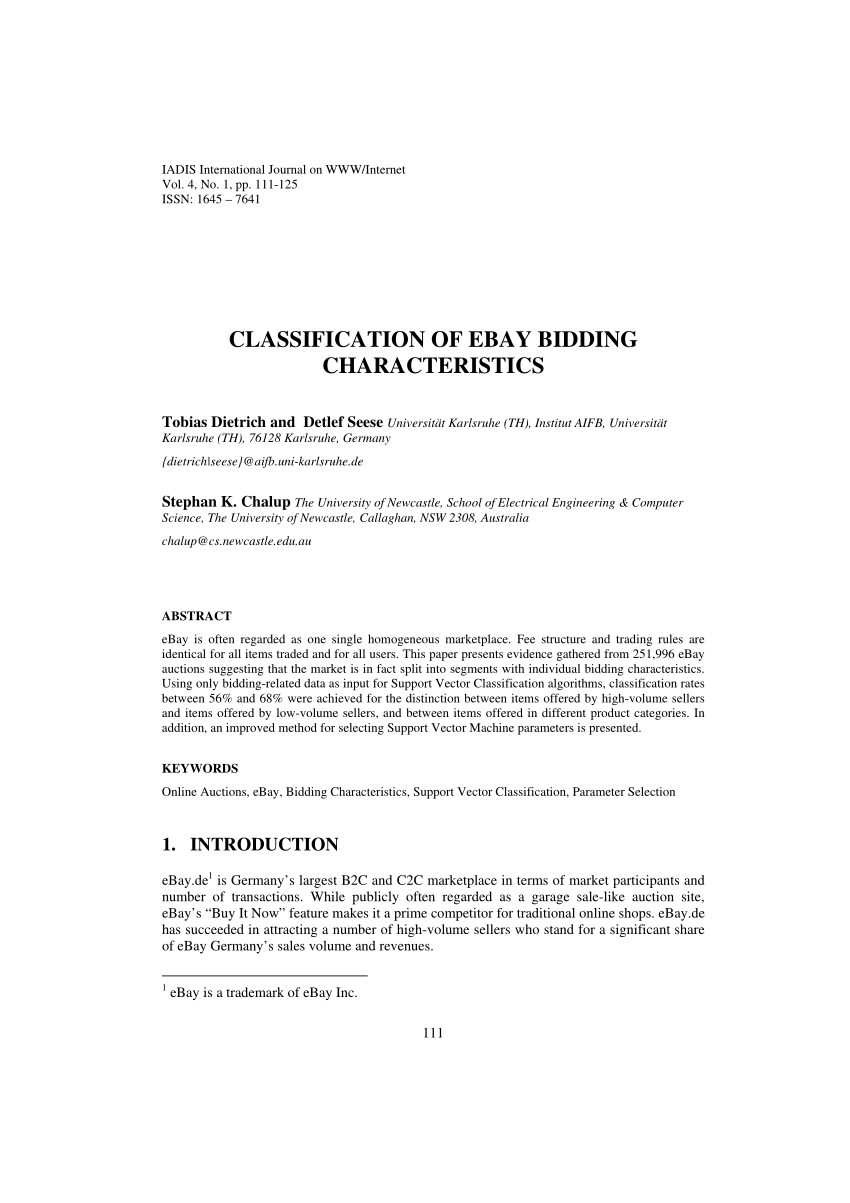
Explain whether each scenario is a classification or regression problem, and indicate whether we are most interested in inference or prediction.
